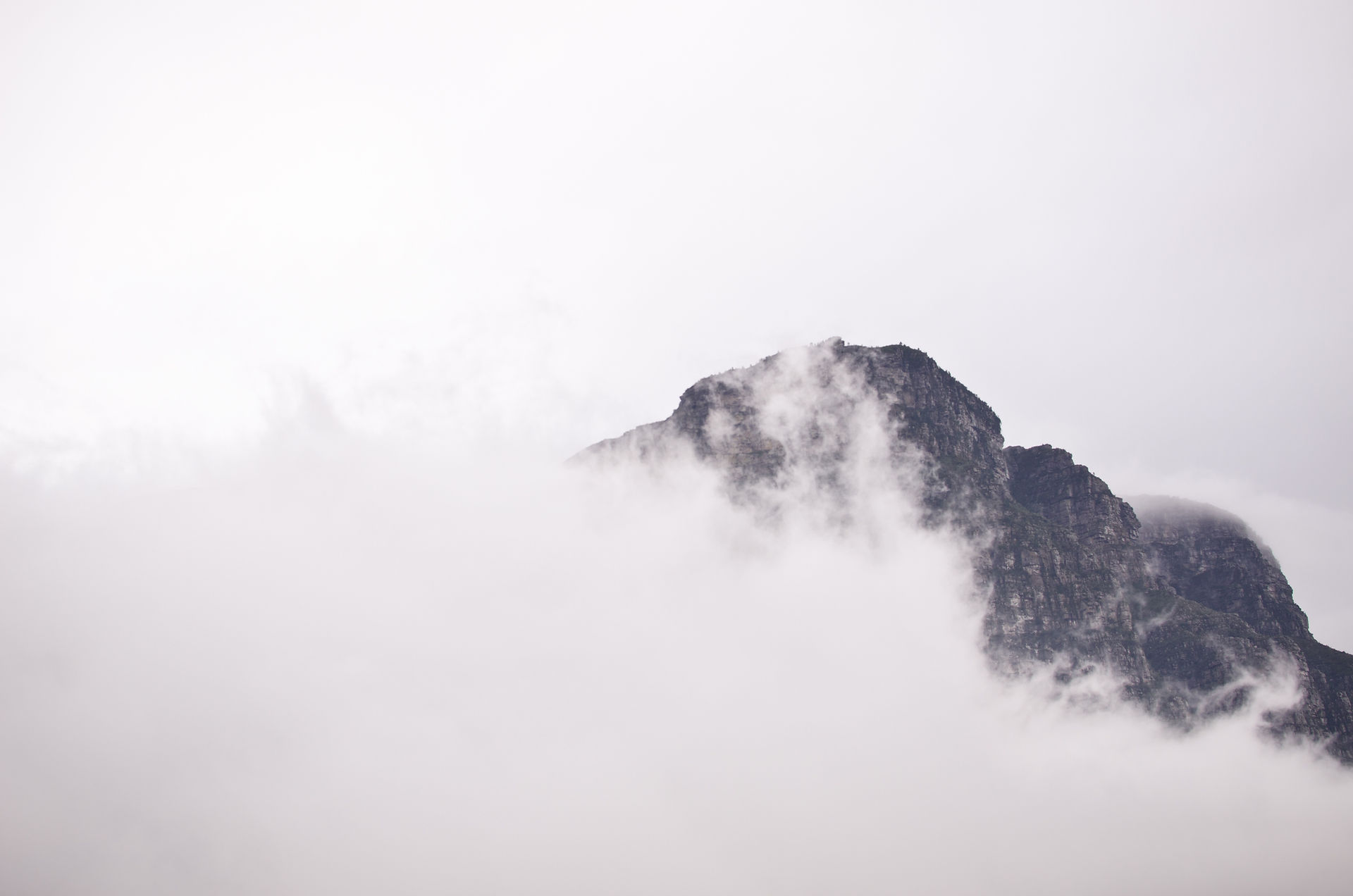
Complex Systems & Control
​
Process Control textbooks often introduce the subject of control through examples. Describing, first, how the temperature of a room or the liquid level in a tank might be controlled, and then moving on to more involved examples that include sequences of tanks, heat exchangers, and mass transfer operations. These examples, and the focus of these texts, are non-complex (process) systems.
By this, I don’t mean that the systems covered in these texts are simple—they may be quite complicated, including many components and having hundreds or even greater numbers of variables. But they do have a certain property that keeps them from being complex. And this is crucial for the application of the control design framework given in these texts: With each of the systems described, there is some clarity around the cause-and-effect relationship between inputs, the underlying components of the system, and the emergent dynamics or output of the system. That is, the effect of the inputs can, with some work, be understood and traced through the system to the outputs that must be controlled.
​
These are the types of systems we know how to control. And we have an almost-formulaic approach for doing so: first, apply first principles and our theoretical understanding of the system to develop a mathematical model of the system dynamics, and then apply mathematical or computational analysis to identify the optimal control strategy (according to that model). There are many examples of this working very well for the types of systems given close attention in process control textbooks.
​
But not all systems have the same character. There is another class of systems that is not fully considered in process control texts; these are complex systems.
​
Complex systems are those featuring a large number of highly interacting components whose
aggregate behavior appears ordered or coherent in some way but, nevertheless, cannot easily be extrapolated from the summation of the individual components and their interactions.
​
​
There are many examples of complex systems at many scales—from self-organizing molecular solutions, to the global climate or the economy. For me, the example that drives home the idea of a complex system is the brain.
The brain is composed of more than 180 billion neuron cells, each of which is connected to as many at 15,000 others. With the brain, it is evident that we cannot consider the activity of each individual neuron and derive the emergent system behavior, as we might for a series of a tanks in a chemical process. Indeed, what emerges from the brain at a macroscopic or collective level, often seems entirely distinct from the underlying components—the emergence of something like consciousness, for example. This is a complex system.
​
Put another way, a complex system is a system for which the components are so numerous and
so intermingled that we lose clarity around cause-and-effect and can no longer accurately derive the
emergent system behavior from our understanding of the system components and any inputs.
​
​
These defining properties—the loss of clarity around cause-and-effect and the emergence of hard-to-explain aggregate behavior—make complex systems fascinating. But the properties are also important to recognize for another reason, one that I am focused on: they disrupt the ability to apply the prevailing, fixed-model-based approach to control design.
And this is salient: complex systems, like the economy or the human body, are certainly some of the most important systems to try to understand and, ultimately, manage or control. Yet, the formal control design frameworks that I see in academic texts do not address such systems.
​
I am interested in researching and developing a new framework for control design—one that can be applied to complex systems.
Complex Systems | {in the context of control}

Human-Inspired Design
The prevailing engineering design framework or paradigm for establishing control can be seen as following two steps: (1) model and (2) calculate.
​
This simple description does not do justice to the many remarkable advances in the theory, tools, and applications; nor does it fairly capture the sophistication of modern modeling and calculation. But it does capture the essence and logic of the underlying control design paradigm: first the system must be understood and described with a precise model, and then calculation can be carried out to determine the best control strategy for the system according to the captured understanding.
​
This strategy is compelling in its logic: don't we need to understand the system and how it works before we can go about determining the appropriate control strategy? Is there even an alternative? What is more, the framework clearly works in many cases—it has been expertly applied to establish very useful control systems in manufacturing, vehicles, and every day appliances.
​
But there is a fault in this default strategy: it can only be applied to systems that submit to precise, upfront mathematical or computation modeling. Complex systems do not fall in that category.
Describing a complex system with a fixed mathematical or computation model that is both detailed enough to accurately capture the global dynamics and also simple enough to be applied for control calculations, is almost always impossibly demanding. This leads to a question that I have been considering:
​
Can we establish control strategies for complex systems without
first being able to fully understand and accurately model their dynamics?
​
Observing human ability might suggest a route. And the specific example that I like, is learning to drive a car.
​
Here, the system dynamics are complicated. Among many other factors, the dynamics of a car must depend on the variable road conditions, dry, wet, icy, etc. These environmental conditions cannot be fully predicted a head of time, nor can their effects be precisely calculated even if they are predicted—this negates the possibility of coming up with a precise, fixed model of the dynamics ahead of time that is accurate and globally predictive and can be used for detailed calculation of control actions to take.
And yet in this context, with a person behind the wheel, it doesn't seem to matter. The control task presents little difficulty for most 15-year-olds: a person intuitively learns to drive a car despite variable road conditions and traffic patterns. This is all done without considering of any formal model or performing detailed calculations upfront.
This observation, and others on human ability, suggest that there is an effective solution or approach for operating in, managing, or controlling complex systems—we just haven't developed that approach in engineering.
Steven Pinker in How the Mind Works puts it this way, "... the engineering challenges that we humans solve as we see and walk and plan and make it through the day are far more challenging than landing on the moon or sequencing the human genome. Nature, once again, has found ingenious solutions that human engineers cannot yet duplicate."
​
That was written prior to 1997. In the time since, we have come a long way. While we are still seemingly just as far from unraveling many of the mysteries of the human mind that lead to our endlessly fascinating and complex abilities, several recent developments should stimulate new interest, enable more advanced formulations that produce results closer to and beyond human abilities, and allow for new practical applications (like those I am interested in).
​
The first notable development is the continued exponential rise of information technology—with many orders of magnitude greater computing power, extensive development in sensors, and near-instantaneous communication capabilities.
The second, is the rapid progression of the field of Machine Learning and the adoption of new ideas, algorithms, and strategies from this field in advanced operations research, computer game playing, and autonomous vehicle control.
​
With these advancements, it is my goal to establish an adaptive or learning framework—along the lines of
what a person accomplishes intuitively when learning to drive—to enable control of certain complex systems.
​
Pursuing an Adaptive/Learning Control Framework

Applications
Agile Pharmaceutical Manufacturing
Closed-Loop Drug Delivery
While the net effect of modern pharmaceuticals is almost certainly positive, pharmaceutical-based treatments continue to face prominent challenges. It is not uncommon to see the Number Needed to Treat (NNT)—roughly, how many patients must be subject to treatment to see one positive outcome—of 5 or higher. And pharmaceuticals almost always come with a list of possible side effects.
​
A common explanation is that drugs are not tailored to individuals and their health-state: to improve efficacy and minimize side effects, the argument goes, pharmaceuticals must be personalized. While this argument for personalized medicine rings true, the commonly proposed solution of tailoring pharmaceutical compounds may be too narrowly focused.
A control-systems perspective highlights a different potential cause of inconsistent efficacy and side effects: pharmaceuticals are currently administered with a fixed, one-size-fits-all strategy—dosing the same fixed amounts of the medicine at regular intervals. And this perspective suggests an alternative way to personalize medicine: medicine could be personalized, applying a tailored administration strategy that uses feedback to deliver just the right amount of the medicine at the right time for each individual patient. In other words, personalizing the administration or delivery of pharmaceuticals, rather than the compounds themselves.
​
This strategy holds promise and is being actively pursued across the pharmaceutical industry and by academics. But, here again, there is an underlying control challenge.
Even for the easiest-to-perceive applications, like management of Diabetes, it has taken decades of dedicated effort to establish appropriate control strategies for closed-loop drug delivery. This development, like tailoring compounds, is too slow and too effortful.
I believe the same reason that I gave for the hindered advancement in agile pharmaceutical manufacturing, might also be slowing down the development of closed-loop drug delivery: the human body is an incredible complex system—applying the default approach to control design is difficult.
​
Instead, we need a different approach. And, again, we can take inspiration from observed human ability.
​
In speaking with individuals afflicted with Type 1 Diabetes, I have found that each has developed a strong sense of the dynamics of their specific glucose metabolism and have intuited insulin dosing schemes that work better than strict adherence to a fixed schedule or formula. The mental models and schemes they use where not developed through a formal, first-principles-type understanding of glucose metabolism, but instead learned over time with observation.
With recent advances in sensors, computation, and machine learning, I believe this same type of strategy might be codified and implemented without a human in the loop. And, I further believe, if that development occurs, closed-loop drug delivery could be expanded beyond Diabetes management to enable personalized and more effective treatment of other debilitating chronic diseases, like cardiovascular disease, arthritis, and cancer.
As with agile manufacturing, It is my hope that a human-inspired control design framework, again built for complex systems, could promote closed-loop drug delivery. And, in doing so, could improve or even save lives.
​
​
​
​
I am enthusiastic about these applications. Have a comments or related opportunities?
For multiple decades, observers and thought leaders in the pharmaceutical industry have noted that manufacturing must become more advanced and agile.
Following on these observations, there has been widespread discussion of continuous manufacturing, process analytical technology (PAT), and automation. And, indeed, many pharmaceutical companies have place significant human and financial resources behind these strategies. But, despite the concerted effort, the industry has not yet experienced a revolution in manufacturing—where progress has been made, it has been slow.
​
One explanation, that many have suggested, is the highly regulated environment. Considering the underlying operations in pharmaceutical manufacturing, however, I would suggest that there has also been a technical challenge that has more directly inhibited progress: Many of the processes in the unit operations for producing pharmaceutics are complex. Crystallization and bio-reactions, for example. And the prevailing approach to design is ill-suited for establishing reliable and flexible control over such operations.
It is my hope that a new control design framework, built for complex systems, could help advance crystallization, reaction, and bio-reaction control, and thereby promote agile pharmaceutical manufacturing.
Research Interests
Control of Complex Systems
Also interested in these topics? Or, have a related opportunity?
current thinking on complex systems, control design, and applications of this research

I currently work as a Sr. Engineer in Synthetic Drug Substance, Pivotal Process Development at Amgen, Inc.
The views expressed in my current research interests, however, are my own;
they have not been endorsed by, nor do they necessarily represent the views of, Amgen.
copyright 2017, Daniel J. Griffin